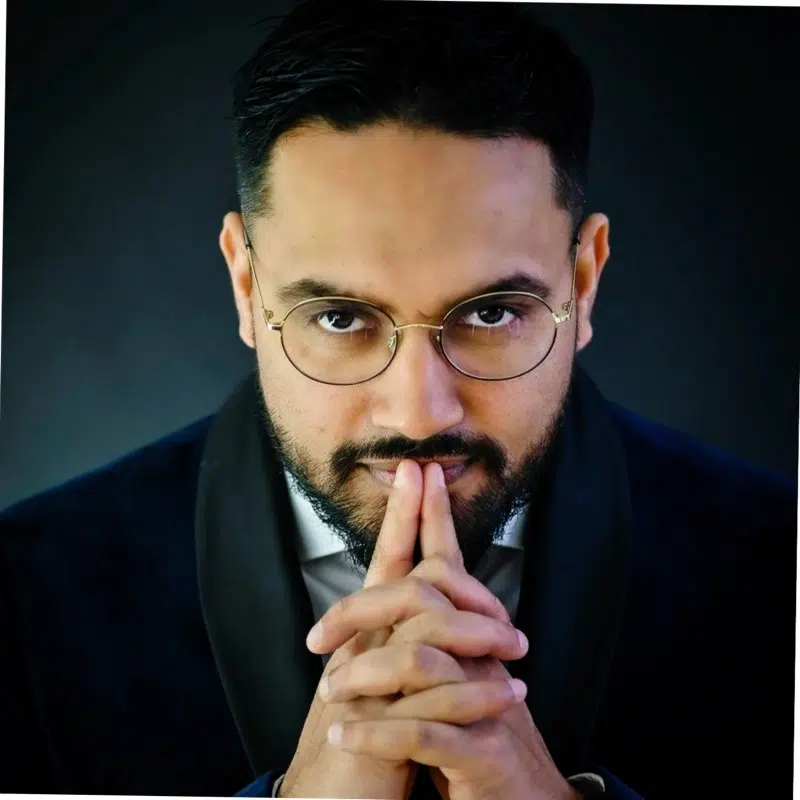
“Our ambition is to become the first pharma company powered by artificial intelligence at scale, giving our people tools and technologies that focus on insights and allow them to make better everyday decisions. … We have just scratched the surface as to how we embrace these disruptive technologies to achieve our ambition of transforming the practice of medicine.”
Paul Hudon, CEO Sanofi
“AI will not replace humans, but humans using AI will.”
Fei-Fei Li, PhD, Professor of Computer Science and
Co-Director of Stanford Institute for Human-Centered AI, Stanford University
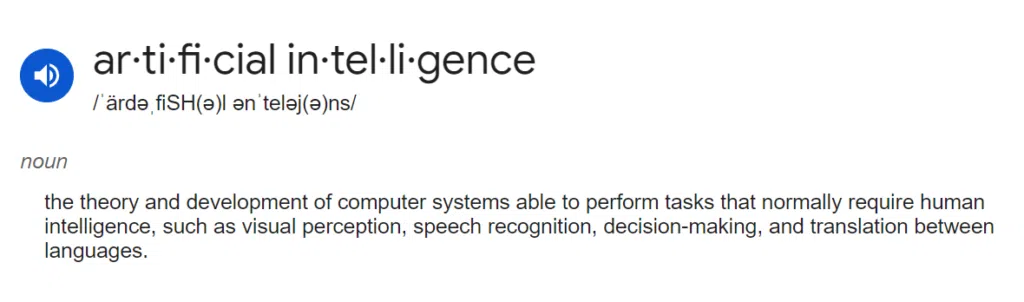
Artificial Intelligence (AI). It’s transforming industries, pharma included.
From machine learning (ML) algorithms to large language models (LLM), deep learning, and the rise of Generative AI (GenAI), AI’s trajectory mirrors—and at times, seems to fuel—the pace of rapid innovation.
Whether you believe the AI hype or not, it’s becoming increasingly impossible for people to avoid AI text, image, audio, and video tools. If you use Google, you’re seeing AI Overviews with every search.
So let’s pause for a moment.
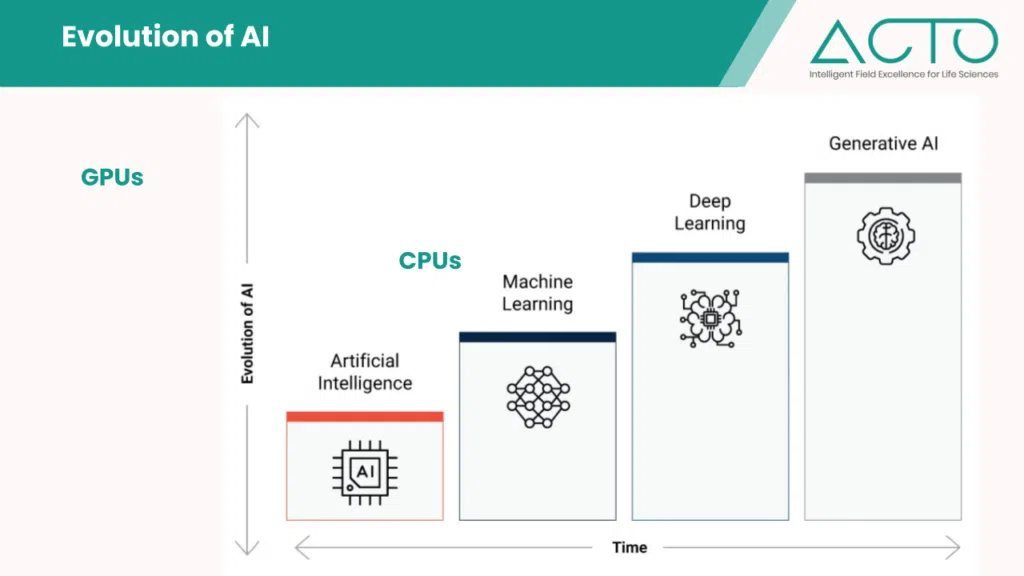
The Dawn of Machine Learning
Machine learning (ML), often hailed as the bedrock of modern AI, began with the Perceptron in 1958. Developed by the American psychologist Frank Rosenblatt, the Perceptron was one of the first machine learning algorithms to use artificial neural networks (a neural network is a computer system modeled on the human brain and nervous system).
The Perceptron was designed to improve the accuracy of computer predictions. The goal was to make it easier for computers to learn from data and to improve upon previous methods. It was basically the first computer to learn new skills by trial and error, using a type of neural network that simulates human thought processes.
ML uses algorithms to analyze large amounts of data, identify patterns, and make predictions. The more data a machine learning system is exposed to, the better it performs. In pharma, ML analyzes vast datasets to identify patterns and predict outcomes in areas such as:
- Drug discovery: ML models accelerate the identification of potential drug candidates by predicting how different compounds will interact with biological targets.
- Predictive analytics: Through ML, pharmaceutical companies can forecast drug demand and optimize supply chain logistics—essential for maintaining market competitiveness.
- Market segmentation: AI algorithms can segment patient populations based on demographics, medical history, and other factors to identify likely responders to a new drug, allowing for targeted marketing campaigns.
- Clinical trial optimization: AI can be used to select optimal patient populations for clinical trials, predict trial outcomes, and improve recruitment strategies.
- Sales force optimization: AI can analyze sales data to identify high-potential sales territories and optimize sales representative interactions with healthcare providers.
- Real-time monitoring: AI can monitor post-market drug safety data to detect potential adverse events and quickly implement necessary interventions.
- Drug repurposing: AI can identify potential new uses for existing drugs by analyzing data on different disease pathways and drug interactions.
The Rise of Large Language Models
Large Language Models (LLMs) are AI models using ML to understand and generate human language. LLMs are trained on large amounts of data, such as text from the internet, and can perform tasks such as text analysis, sentiment analysis, and language translation. These models can generate human-like text and understand context far beyond earlier AI capabilities, including:
- Research and development: LLMs assist researchers by quickly processing and summarizing vast bodies of literature, leading to faster insights and innovations.
- Patient interaction: Automating patient queries and support through LLM-driven chatbots enhances customer service and engagement.
Deep Learning
Deep learning, a subset of ML, employs neural networks with multiple layers to model complex patterns in data. This technology drives significant advances in image and speech recognition and is now making waves in pharmaceuticals in areas such as:
- Medical imaging: Deep learning algorithms enhance the accuracy of diagnostic imaging, reducing human error and speeding up diagnosis.
- Genomics: Analyzing genetic data with deep learning helps identify genetic markers of diseases, paving the way for personalized medicine approaches.
Generative AI
Generative AI (GenAI) refers to systems capable of creating new content, such as images, music, and text. In pharmaceuticals, GenAI is opening new frontiers such as:
- Novel drug design: GenAI can generate entirely new molecular structures, offering a plethora of possibilities for novel drug candidates.
- Clinical trials simulation: Simulating clinical trials with GenAI reduces costs and accelerates the time-to-market for new therapies.
- Field rep coaching and performance: GenAI is the basis for virtual assistants who provide answers derived exclusively from company-approved content, so reps receive the most accurate and up-to-date information.
The history of AI in biopharma is more than a technological evolution—it’s a paradigm shift. Staying informed about AI and its applications is crucial to leveraging its full potential.
In the near future, AI solutions promise to reshape the landscape, offering unprecedented opportunities for innovation and efficiency. Now is the time to understand and invest in AI-powered solutions that help to maintain a competitive edge as well as bring drugs to market faster to patients waiting in need.
Learn more about LAICA RepAssist by ACTO – the only AI Knowledge Assistant built for pharma field reps!
This blog is the first in a series of posts from ACTO’s CTO that will discuss what:
- Pharma people should know about AI,
- AI myths that need debunking, and
- Use cases demonstrating how AI can bridge the “human-touch” gap, so field reps can be more competent and confident in face-to-face HCP interactions.
Look for the next blog in the series on December 3rd.