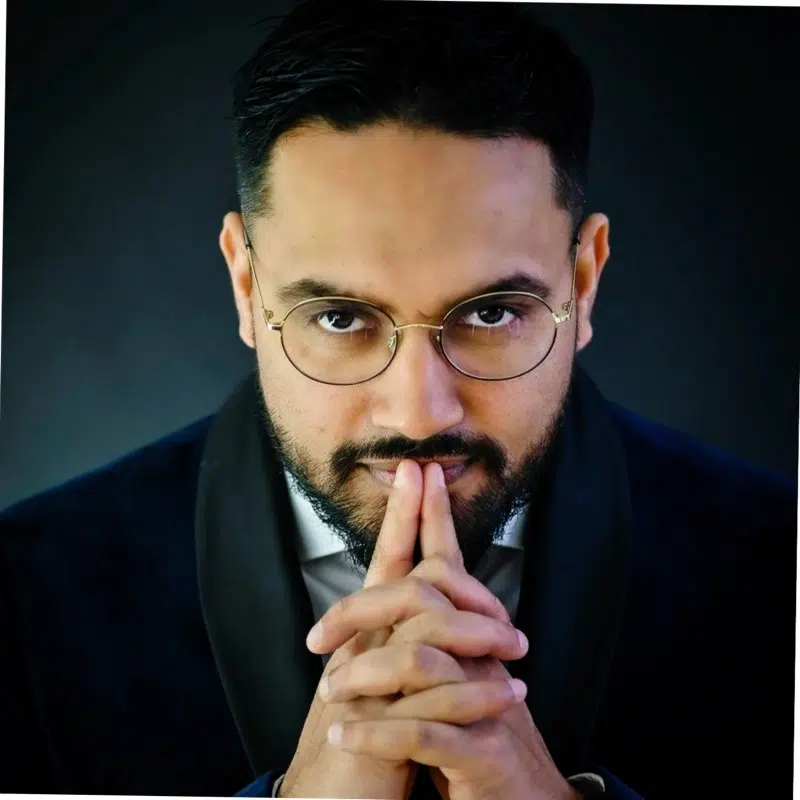
Part 2 – Our first blog covered the ABCs of Artificial Intelligence (AI) and AI’s evolution. This post discusses key issues pharma executives must consider to ensure responsible, compliant, and successful AI implementation within their organizations.
The stakes for AI adoption in the pharmaceutical industry have never been higher. A survey of healthcare and life science companies found that 93% of these companies expect to increase their spend in 2025. In addition, 33% of them are currently piloting different solutions in sales, and 44% plan to adopt AI in sales in the next 24 months.
Yet, the critical question remains: How can pharmaceutical companies ensure responsible and compliant AI implementation while maximizing its potential for field teams? How can they ensure that these solutions meet the stringent requirements of the biopharma industry? What issues should they consider to maximize the impact of AI solutions not just in sales but also companywide?
The Foundation: Governance and Security
Successful AI implementation begins with strong platform governance and security standards. Organizations must:
- Develop comprehensive ethical guidelines that align with industry regulations
- Deploy multi-layered security protocols to protect sensitive data
- Create transparent AI decision-making processes
- Conduct regular stakeholder impact assessments
To develop such a strong foundation, leading pharmaceutical companies are establishing dedicated AI Councils to coordinate AI initiatives across their organizations. These councils bring together diverse expertise from scientists and researchers, data analysts and technology specialists, regulatory compliance experts, as well as commercial operations leaders. Such cross-functional collaboration ensures consistent implementation from drug development through commercialization.
The Foundational Pillars
ACTO believes in implementing four foundational pillars that will ensure responsible AI solutions that address pharma’s highly regulated parameters, while providing value. They are:
- Building A Strong Foundation: Data Security and Privacy
- Maintaining Robust Standards and Compliance
- Ensuring Data Integrity: Accuracy and Consistency
- Asserting Active Human Oversight and Robust Governance
1. Building A Strong Foundation: Data Security and Privacy
When implementing AI solutions, ensuring proper data anonymization is non-negotiable. Since the biopharmaceutical industry deals with sensitive information daily, all data must be thoroughly scrubbed of personal identifiable information (PI) before being used for AI model training or fine-tuning. This protects privacy while allowing organizations to leverage valuable insights from their data.
In addition, content used for AI applications, particularly in knowledge bases for Retrieval-Augmented Generation (RAG), must maintain strict residency requirements. RAG is an AI framework that combines information retrieval systems with large language models (LLMs) to generate more accurate and relevant text. It uses search algorithms to query external data, such as knowledge bases, web pages, and databases.
Keeping content within an environment that controls access to network-based content and services (i.e., a “walled garden” of sorts), ensures data sovereignty and compliance with regional regulations. This approach provides better control over data access and security.
2. Maintaining Robust Standards and Compliance
Pharma must understand that regular maintenance of security standards through certifications that ensure security and privacy (SOC 2 Type ll and/or GDPR), compliance (FDA 21 CFR Part 11), as well as control (approved by MLR) is much more than just about checking boxes. These frameworks provide essential guidelines for:
- Maintaining traceability of AI decisions
- Ensuring accountability in AI systems
- Supporting explainability of AI outputs
- Meeting a range of regulatory requirements specific to the pharmaceutical industry
3. Ensuring Data Integrity: Accuracy and Consistency
The main priority to ensure data integrity is the proper contextualization of AI systems so they provide accurate outputs. Using content deployment and access mechanisms ensures that AI models draw from appropriate, relevant information sources. This requires:
- Careful curation of knowledge bases
- Implementation of proper content access controls
- Regular updates to maintain information accuracy
- Clear governance structures for content management
Another concern around ensuring data integrity is preventing hallucinations, a massive challenge in any AI implementation. Hallucinations are instances where AI generates plausible but incorrect information.
Such a solution involves a multi-faceted approach:
- Implementing RAG systems with rigorous relevancy training
- Conducting thorough pilot implementation phases
- Performing iterative fine-tuning based on feedback
- Following the principle that “no answer is better than a less ranked answer”
4. Asserting Active Human Oversight and Robust Governance
While AI offers powerful capabilities, human oversight remains essential. Organizations must establish:
- Clear protocols for monitoring AI model outputs
- Audits of RAG content governance
- Defined access control mechanisms
- Ongoing training programs for staff managing AI systems
Remember the “garbage in / garbage out” axiom. The quality of AI outputs directly correlates with the quality of input content. Establishing robust content governance ensures:
- Accurate and up-to-date information in knowledge bases
- Proper vetting of information sources
- Regular content reviews and updates
- Clear and transparent accountability for content accuracy
For pharmaceutical executives, implementing AI isn’t just about adopting new technology – it’s about doing so responsibly and effectively. By focusing on data security, maintaining high standards, ensuring accuracy, and implementing proper governance, organizations can harness AI’s potential while minimizing risks.The key is to remember that successful AI implementation requires a balanced approach between innovation and responsibility.
The future of pharmaceutical AI lies not just in the sophistication of the models, but in how well organizations can govern, control, and apply these powerful tools in ways that benefit stakeholders, such as field teams, while maintaining the highest standards of security and ethics.
Learn more about how to leverage AI knowledge assistants to help your reps with messaging and information while in the field.